Greasing the Groove with Physics-informed AI and Blockchain in Heavy Industries
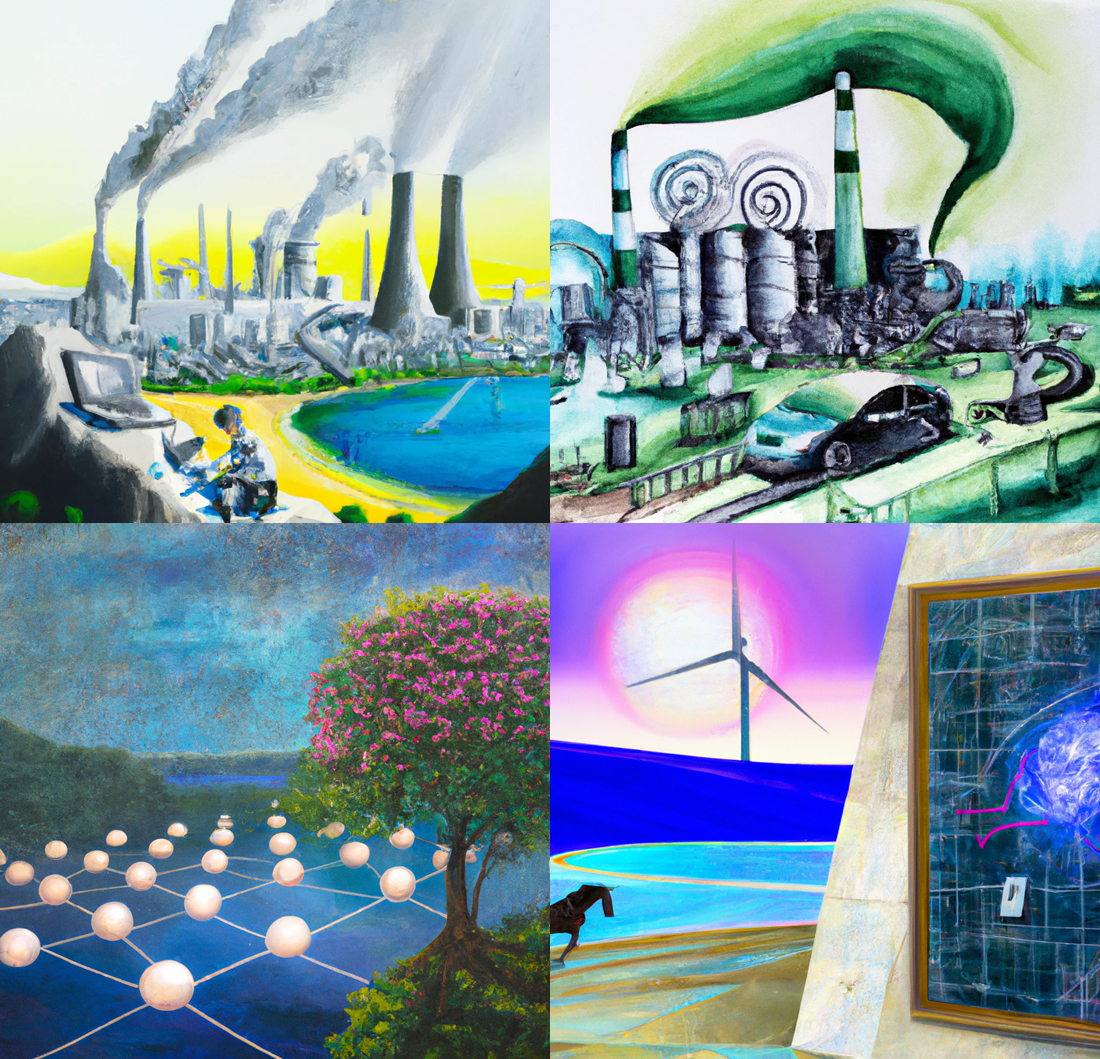
The future of systemic and autonomous artificial intelligence (AI) is shrouded in potential but equally fraught with uncertainty. Recent decades have shown us that AI systems can accurately model complex systems and quantitatively inform us, allegorically, that “the apple resides precisely at the top of the tree”. Knowing where this allegorical apple resides offers some value, but knowing why it resides there and how we can reach it would then bridge this crucial ravine and integrate such data-driven models into established knowledge frameworks.
Even if we manage to integrate such Internet of Things (IoT)-centric concepts and AI systems across our sustainable technology landscape, there are still large challenges pertaining to establishing data-privacy, enhancing connectivity and bandwidth, concurrent collaborative learning and upgrading workforce competencies that are intrinsically linked to this progression1˒2. But despite the many unknowns about the future, the potential opportunities of AI-driven systems to enable decision support and resolve the management of complexity are too great to overlook3. As artificial intelligence of things (AIoT) establishes autonomy in understanding and responding to the complexities of the industrial world, its capabilities will continue to increase, which is likely to result in increased economic value creation.
Please join me in this curious narrative as we unpack some of the ways that AIoT, Blockchain Technology, Physics-Informed Machine Learning and creativity can aid us in making better informed technology decisions and optimize efficiencies within this sustainable future state. Much like my other written articles, there will be an eclectic smattering of allegory and analogies. To avoid the risks of being too generic, I will also select a suitable chaperone that takes us into this industrial topic; in this case steel. Let us first define our landscape…
The Trees (AIoT) and The Pollinators (Blockchain) in the Meadow of Heavy Industries
Industries account for about 30% of total final energy consumption worldwide and about 20% of global CO2 emissions. Steel, cement, and chemicals are the top three emitting industries and are among the most difficult to decarbonize, owing to technical factors like the need for very high heat and process emissions of carbon dioxide, and economic factors including low profit margins, capital intensity, long asset life, and trade exposure4.
Source5.
It is clear that there will be steep technological learning curves associated with the transition to lower-carbon technologies, which will bring about a short-term economic burden to the industrial early adopters6. Policymakers, the finance sector and other stakeholders need to work together to create a stable framework to encourage wider investment in low-carbon production, and reduce the price premium so that consumers buy greener alternatives, such as green steel products5. For segments such as material commodities, it is clear from the figure above that Scope 1 and 2 emissions constitute a majority of the total emission footprint, which merit radical solutions. Even within the green steel landscape, there have been some noteworthy developments, such as SSAB’s hydrogen-based HYBRIT project7 and Boston Metal’s molten oxide electrolysis8, which also lends itself to more abundant lower grade iron ore9 and modular mini-mill operations. But knowing how to make the most informed technology decisions still remains a complex and systemic matter2. For example, in the case of steel production in Sweden, the hydrogen-based steel production HYBRIT project alone will require an additional 15 TWh/year of electricity capacity from the national grid, which also highlights the systemic nature of such endeavors10. This will naturally demand increased grid capacity and smart grid management, whereby AIoT is primed to operate11. Fortunately, for places like Sweden and Canada, renewable energy is relatively cost-effective, particularly hydropower, due to abundant hydropower resources that can be exploited at large scales, no fuel costs and through electricity pricing policies4˒12. However, if we now consider this from a global perspective; we realize the immense complexity in this relational and contractual future whereby green energy will be the flowering nectar in this digital meadow of smart systems. This brings us to one of the most promising and important pollinators within this space; blockchain technology13.
Blockchain technology has the potential to revolutionize the way that smart grids and heavy industries operate, particularly in the areas of supply chain management, renewable energy and energy certificates14. In the future, it is likely that blockchain-based systems will be used to track the production and consumption of electricity in smart grids13, as well as to facilitate the trading of renewable energy credits, which will be inherently integrated into future low-carbon heavy industries. Additionally, blockchain could also be used to create a transparent and secure system for tracking and certifying the offsetting of carbon emissions within heavy industries; by securely integrating the involvement of renewable energy producers (such as local solar farms)15. This could help to create incentives for the energy industry to rapidly evolve into a federation of local smart energy girds which would eventually reduce the economic burden on the energy-intensive users; the heavy industries.
Here are a few examples of how blockchain technology can support smart grids within heavy industries:
Supply chain tracking: Blockchain can be used to track the origin and movement of raw materials and commodities, enabling more transparency and traceability in the supply chain14. This can help improve the efficiency and sustainability of the supply chain, as well as enhance the security and integrity of the data.
Trading and settlement: Blockchain can be used to facilitate the trading and settlement of raw materials and commodities, by automating and streamlining the process and reducing the need for intermediaries16. This can help reduce the costs and risks associated with trading, as well as increase the speed and accuracy of transactions.
Energy trading: Blockchain can be used to enable peer-to-peer (P2P) energy trading, allowing individuals and businesses to buy and sell excess renewable energy directly with one another17. This can help increase the adoption of renewable energy and improve the efficiency of the energy grid15.
Quality assurance: Blockchain can be used to store data on the quality and safety of raw materials and commodities, helping to ensure that they meet regulatory standards and customer requirements. This can help improve the reliability and trustworthiness of the supply chain18.
This ultimately brings us to a crucial point that illustrates the spectrum of creativity required in establishing this low carbon future; we must think systemically and imaginatively about solutions that best harmonize with a given locality and our environment, integrating both the “old” and the “new”.
“The function of imagination is not to make strange things settled, so much as to make settled things strange; not so much to make wonders facts as to make facts wonders.” - G. K. Chesterton
A bag of sand is as pragmatic as a full-stop and could in many cases, masquerade as a mascot for something factual. But even within the simplicity of sand, there resides a wondrous capacity to store energy and complement the intermittent-nature of renewable energy sources19˒20. But before we all head to the beach with spades in hand, there are also other novel solutions which bring wonder into the simplest of concepts. Much like buying a house, location dictates matters greatly. EVRAZ Pueblo in North America have capitalized on the high amount of annual sunshine they experience in Colorado, whereby an on-site solar farm will offset about 90% of the steelworks electricity needs21. From the cradle of steel production to the grave of metal recycling, the harnessing of solar energy also holds great promise in enabling sustainable processes within the circular product economy of metals22. The list of novel solutions here is far from static and it will be imperative for us to devise ways to integrate such distributed and federated technologies into these smart heavy industries15. But what challenges reside within this transition?
Growing and Protecting Your Smart Data Meadow
The use of AI has made great progress over the past decade and has proven highly successful in both predicting and prescribing complex behavior within smart manufacturing, such as steelmaking23˒24˒25˒26. Overall, the use of AI within raw materials has the potential to improve efficiency, reduce costs, and increase sustainability by optimizing the production process and support integrated smart energy systems. However, there are several barriers to the wider adoption of artificial intelligence (AI) and machine learning in the hard-to-abate industries, much of which are not exclusive to the steel industry. These can be listed as:
- Lack of knowledge and skilled personnel: There is a shortage of skilled personnel with combined expertise in AI (specifically in “opaque” deep learning methods) and domain specific knowledge, which can make it difficult for heavy industries to find and hire the talent they need27. The renewable energy sector tends to be more labor-intensive than conventional energy sectors and therefore, the realization of high labor productivity will require a reciprocal technology intensity which reinforces the need for upskilling the workforce28.
The high-level
ontology of explainable artificial intelligence approaches . Source29.
High upfront costs: Implementing AI and machine learning systems can be expensive, especially for small and medium-sized businesses. When a massive number of sensors is utilized, putting a high computational burden on every sensor would be very costly. Compounding these costs with other necessary decarbonization costs such as automation and electrification contextualizes this particular challenge. One way to circumvent this is to establish cloud computing, whereby lower cost sensors (with adequate latency and bandwidth) and low cost nodes (such as recycled and repurposed smartphones30) can alleviate the consolidation and computational burden to the cloud31.
Data and complexity issues: Many heavy industries struggle to collect and manage the data required to train and utilize complex machine learning systems, which can be a significant barrier to adoption. With the proliferation of sensors and devices that generate data, there is an increasing need for robust and explainable methods that can analyze and make sense of this data32.
Model explainability
vs. model performance for widely used machine learning and deep learning
algorithms. The ideal solution should have both high explainability and
high performance. Source33.
Regulatory and legal considerations: There are often regulatory and legal considerations to take into account when implementing AI and machine learning systems, which can be complex and time-consuming34.
Ethical and data privacy concerns: There are also ethical concerns surrounding the use of AI and machine learning, which can be a barrier to realizing sustainability goals within heavy industries35. One solution to this critical issue has been federated machine learning. This is a technique for training machine learning models on decentralized data, such as data that is stored on users’ devices or on multiple remote servers. One of the main benefits of federated learning is that it allows organizations to build machine learning models without having to access the data directly and thus maintain data confidentiality36. This can be particularly useful for addressing data-sensitive sectors such as healthcare37, as it allows organizations to build models without having to handle sensitive patient data.
Federated learning, explainable AI, blockchain and synthetic data are all techniques that can be used to build machine learning models that address most of the challenges listed above. In my own experience within the world of AI, the whole is often greater than the sum of its constituents, and here is no exception. Here are some ways in which these techniques can be combined:
Why is the apple at the top of the tree? Explainable AI techniques can be used to provide insight into the decision-making process of federated learning models38. For example, Shapley values, which is a method from a well-established coalitional game theory, tells us how to fairly distribute the “payout” among the features of a particular prediction system39. This can be useful for ensuring that the model is making decisions based on relevant and appropriate features, and for identifying any potential bias in the model40. Accumulated Local Effects (ALE) describes how features influence the prediction of a machine learning model on average and is a method that I have found to be particularly effective. What makes this approach particularly pertinent for systemic production in heavy industries (like steelmaking) is that this method can correctly interpret the influence of correlated variables (such as different stages on a rolling line), unlike Partial Dependence Plots (PDP)41.
Synthetic data, another collaborative meadow pollinator: Synthetic data is a type of artificial data that is generated by a machine learning model. It can be used to train machine learning models in an ethical and privacy-preserving manner, as the synthetic data does not contain any real personal data42. Synthetic data can be particularly useful in the context of federated learning, as it allows organizations to train models without having to access real data directly.
Enhance transfer learning through privacy-proof grafting: Federated learning with synthetic data augmentation: synthetic data can be used to augment the training data in a federated learning setting. This can be done by generating synthetic data samples at a central server, and using them to augment the global model. This learned model can then be transferred to the participating devices, and fine-tuned using federated learning43.
By combining federated learning with explainable AI and synthetic data, organizations can build machine learning models that are both accurate and privacy-preserving. However, when capital expenditure limits the ability to upgrade the production technology, or the realization of IoT enabled process methods, what are our options when dealing with older and more nuanced traditional methods? Could the we predict the extent of grain refinement from the hammer blows of a blacksmith?
Greasing the Groove with Physics-Informed Machine Learning
Attribution44.
Deep Learning as the state-of-the-art AI technique has its roots in the emulation of the human brain. There are also some similarities between machine learning neural networks and the pathway forms between human muscles and the nervous system. This biological pathway was famously coined as “greasing the groove” by Pavel Tsatsouline45, which reflects the adaptive efficiency of neuromuscular motor patterns through processes such as neuronal myelination. Both involve the processing and transmission of information through a network of interconnected nodes, and both involve the use of algorithms to analyze and interpret data. Overall, while there are some similarities between machine learning neural networks and the pathway forms between human muscles and the human nervous system, there are also significant differences. The human nervous system is much more complex and sophisticated than any artificial system, with billions of interconnected neurons and a wide range of specialized functions. One notable difference is the embedded knowledge frameworks within the human mind, which serves to interpret and ultimately root model results into first-principles knowledge. This lack of established first-principles knowledge framework and interpretable linkage to model results is the “Achilles heel” to current machine learning approaches38.
An overview on some of my AIoT consulting work (e.g. creation of the Vanalytica solution - https://www.vantage-alloys.com/vanalytica) and experiences with physics-informed machine learning. Approach is industrially agnostic, and can be applied to several industrial domains.
One type of machine learning algorithm that is gaining attention is physics-informed neural networks (PINN). These algorithms are designed to incorporate physical principles and constraints into the learning process, which allows them to make more accurate predictions and decisions46. This is particularly useful in fields such as engineering and materials science, where the behavior of physical systems is governed by complex equations and laws47. For example, PINNs could be used to accurately predict and prescribe performance within renewable energy systems that are data-constrained (not fully IoT saturated), such as accurately modelling battery systems48, prescribing nanophotonics within solar panels49 or optimizing wind turbines materials50. By incorporating physical principles and constraints into the learning process, these algorithms can identify materials with improved performance and durability, which could lead to more efficient and cost-effective renewable energy systems.
Another way that explainable physics-informed neural networks could contribute to the decarbonization of hard-to-abate industries is by helping to optimize the design and operation of industrial processes and systems. These algorithms could be used to analyze data from sensors and other sources to identify ways to improve the efficiency and reduce the carbon intensity of industrial operations. For example, these algorithms could be used to identify opportunities for performance enhancement, energy conservation and waste reduction within additively manufactured materials51.
Outlook - Unlocking New Paradigms
Overall, the future of machine learning and artificial intelligence looks promising indeed, with the potential to revolutionize many different industries and applications. By leveraging the power of data and algorithms, these technologies have the potential to drive significant improvements in safety, efficiency, and performance in a wide range of fields. Much like the dualistic essence of novelty and simplicity within a sand-battery20; empowered AIoT devices convert traditional machines into smart behavior devices31. By analyzing large datasets and incorporating physical principles and constraints into the learning process, these algorithms can identify patterns and trends that may not be immediately apparent to human analysts. This could help to identify areas where carbon reduction efforts would be most impactful, and help to prioritize those efforts based on their potential to reduce greenhouse gas emissions.
Overall, explainable and physics-informed neural networks within IoT-centric systems have the potential to play a significant role in the decarbonization and sustainability of hard-to-abate industries. By enabling the development of new materials and technologies, optimizing the design and operation of industrial processes and systems, and helping to identify and prioritize carbon reduction opportunities, these algorithms could help to drive significant improvements in the environmental performance of these sectors.
Thanks for taking the time to read this article and Godspeed. /Aaron